
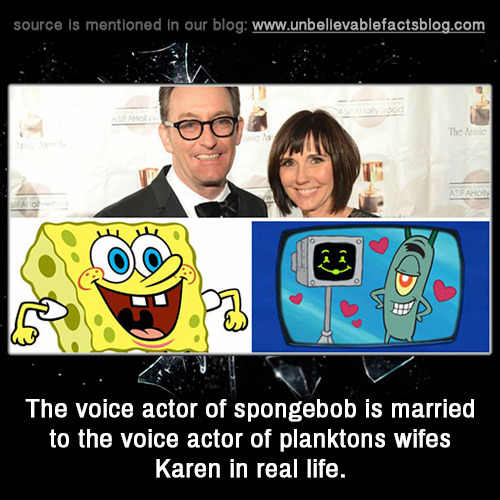


ENGINEER VOICEY ACTOR FREE
In each case, unit costs drop as volume of usage increases, and all the vendors offer a fairly generous free tier before pricing kicks in. Having deprecated their concatenative voices, Microsoft and IBM now appear to offer only neural TTS, at US$16 per million characters and US$20 per million characters, respectively, although IBM’s charging structure actually makes it cheaper than the others at lower volumes. Google price-matches with WaveNet voices at US$16 per million characters and standard voices at US$4 per million characters. Many vendors also provide what they refer to as “standard” voices that use lesser-quality concatenative synthesis at a lower cost.įor example, at the time of writing, Amazon’s Polly will generate synthetic speech for you at a cost of US$16 per million input characters for neural voices you can also use standard concatenative voices at US$4 per million input characters. Today, the state-of-the-art is exemplified by synthesis using deep-learning based models, also commonly referred to as neural TTS, with output that is characterized by natural-sounding changes to pitch, rate, pronunciation, and inflection. Most of the big tech players who offer a suite of cloud-based NLP APIs include text-to-speech in their portfolios. If you’re a developer building an application that needs a text-to-speech capability, you've never had it so good. So, given the advances made over the past few years, what does the commercial voice synthesis market look like today? In this post, we look at the applications of the technology that are enticing investors and aiming to generate revenue, and identify the companies that are making news. As well as providing a noticeable increase in the quality and naturalness of the voice output that can be produced, these have opened the door to a variety of new voice synthesis applications built on deep-learning techniques. The years since have seen the development of a wide range of deep-learning architectures for speech synthesis. Things stepped up a notch with DeepMind’s 2016 introduction of WaveNet, the first of the deep-learning based approaches to speech synthesis. However, by the early 2000s, it was good enough to field telephony-based spoken language dialog systems whose conversational contributions weren't particularly offensive to the ears. The outputs from early applications based on formant synthesis sounded too artificial to be mistaken for human speech and were generally criticised as sounding “robotic.” Subsequent products based on unit concatenation dramatically increased the naturalness of the synthesized speech-but still not enough to make it indistinguishable from real human speech, especially when uttering more than a few sentences in sequence. In the modern era, early attempts at computer-based speech synthesis were already appearing in the 1960s and 1970s, and the 1980s saw the arrival of the DECtalk system, familiar to many as the voice of Stephen Hawking. Humans have been fascinated by the idea of making machines sound like humans for quite a long time, going at least as far back as Wolfgang von Kempelen’s mechanical experiments in the second half of the 18th century.
